
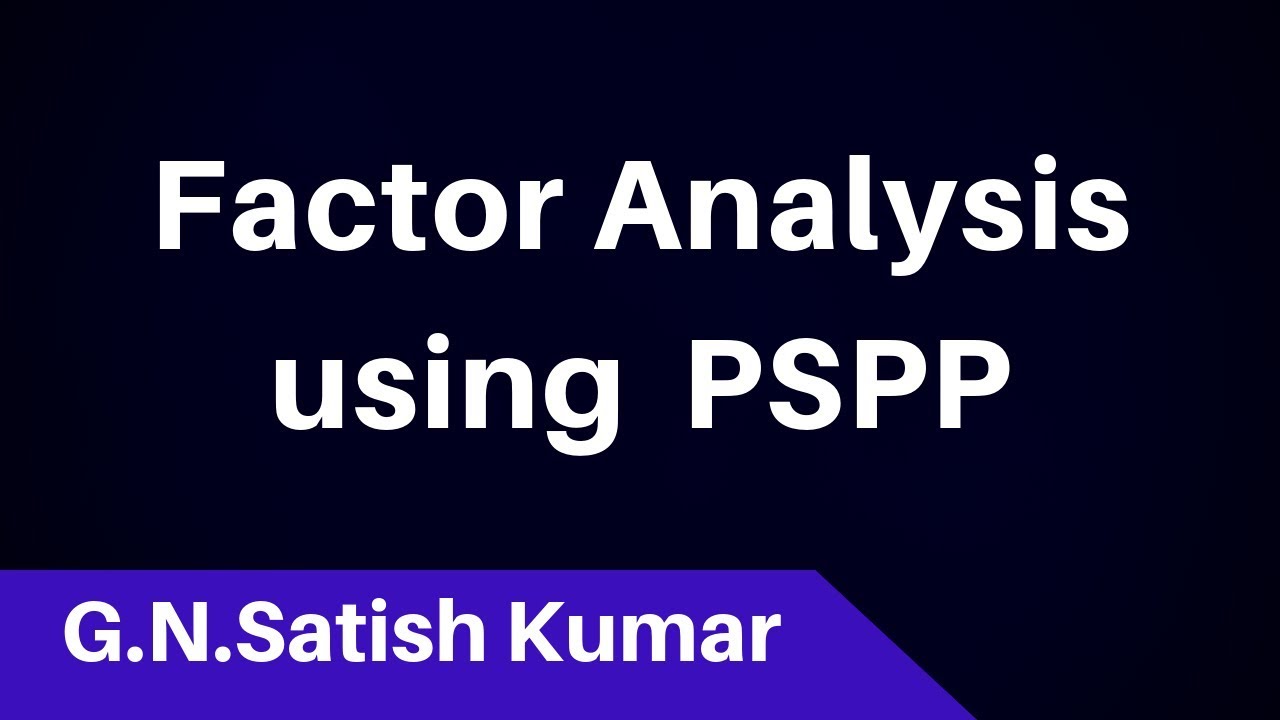
This could also happen due to the sample/subject dropping out of the study for reasons other than death, or some other loss to followup. This happens when you track the sample/subject through the end of the study and the event never occurs. The curve is horizontal over periods where no event occurs, then drops vertically corresponding to a change in the survival function at each time an event occurs.Ĭensoring is a type of missing data problem unique to survival analysis. It’s a step function illustrating the cumulative survival probability over time.
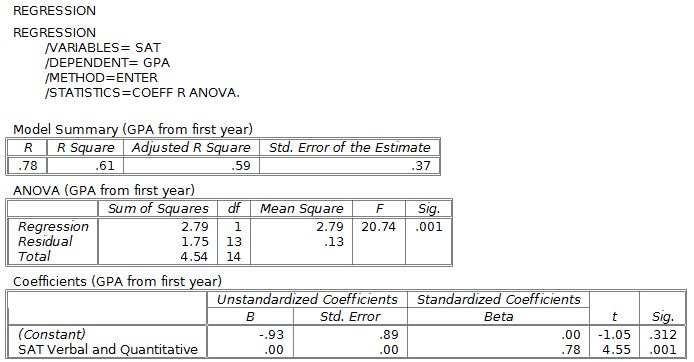
The Kaplan-Meier curve illustrates the survival function. \(S\) is a probability, so \(0 \leq S(t) \leq 1\), since survival times are always positive ( \(T \geq 0\)). It looks like this, where \(T\) is the time of death, and \(Pr(T>t)\) is the probability that the time of death is greater than some time \(t\). It’s the probability that the event (e.g., death) hasn’t occured yet. The survival function, is the probability an individual survives (or, the probability that the event of interest does not occur) up to and including time t. The cumulative hazard is the total hazard experienced up to time t.
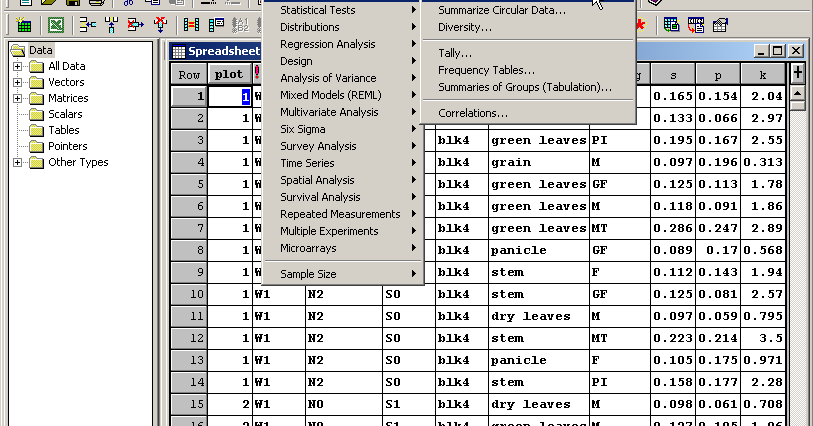
Survival analysis doesn’t assume the hazard is constant over time. The hazard is the instantaneous event (death) rate at a particular time point t. Generally, survival analysis lets you model the time until an event occurs, 1 or compare the time-to-event between different groups, or how time-to-event correlates with quantitative variables. Survival analysis lets you analyze the rates of occurrence of events over time, without assuming the rates are constant. Or, recurrence rate of different cancers varies highly over time, and depends on tumor genetics, treatment, and other environmental factors. For example: the risk of death after heart surgery is highest immediately post-op, decreases as the patient recovers, then rises slowly again as the patient ages. In this kind of analysis you implicitly assume that the rates are constant over the period of the study, or as defined by the different groups you defined.īut, in longitudinal studies where you track samples or subjects from one time point (e.g., entry into a study, diagnosis, start of a treatment) until you observe some outcome event (e.g., death, onset of disease, relapse), it doesn’t make sense to assume the rates are constant. For example, we looked at how the diabetes rate differed between males and females. In the class on essential statistics we covered basic categorical data analysis – comparing proportions (risks, rates, etc) between different groups using a chi-square or fisher exact test, or logistic regression.
